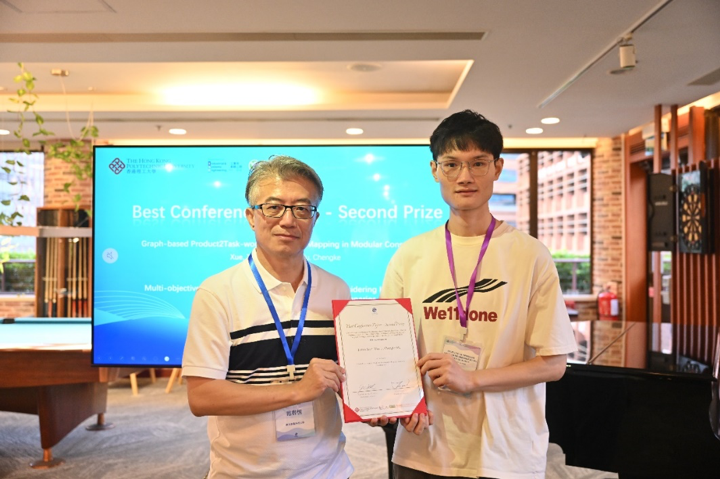
Award |CSIE2024
Congratulations to XUE Jintao from the CI3 lab for winning the Best Conference Paper Second Prize at the Annual International Conference for Chinese Scholars in Industrial Engineering.
A critical stage before work package generation in modular construction (MC) is product2task mapping. However, the ability to perform complex reasoning and the richness of semantic information in mapped tasks still need improvement. This paper addresses the abovementioned problems through a graph-based product2task-worker-machine mapping (P2TWM) framework (G-P2TWM). The framework first represents the data, including tasks, products, workers, and machines, as knowledge graphs (KGs). Then, a novel heterogeneous graph neural network for mapping in MC (HGN-MC) is employed to seamlessly extract KG data and adaptively map the products to the corresponding tasks, workers, and machines (e.g., rebar fixing, structural steel welder, and rebar bending machine), thereby enriching task-level information for the downstream process in MC (e.g., work package generation and production scheduling). The proposed G-P2TWM framework also supports flexibly adding additional heterogeneous information (e.g., spatial information) as graph nodes to enhance mapping performance. Experiments demonstrate that our method can produce complex knowledge reasoning with high performance.